Quantitative or systematic investing has evolved rapidly in recent years, particularly as the opportunities provided by artificial intelligence and Machine Learning transition from the laboratory through into client portfolios. At Man AHL (‘AHL’) we have been successfully trading Machine Learning based systems in our multi-strategy client portfolio since early 2014, and Machine Learning is a core area of research effort both within AHL and at the Oxford-Man Institute, our unique collaboration with the University of Oxford.
So what is Machine Learning? Machine Learning is a catch-all name for a range of applied practical algorithms that can identify repeatable patterns and relationships within observed data, and importantly can do so without having to be told explicitly what kind of patterns and relationships to look for – the algorithms work that out for themselves. This is what distinguishes Machine Learning from more traditional data analysis techniques. Such algorithms arise in computer science, information engineering, statistics and various mathematical disciplines, so Machine Learning is best thought of as a hybrid discipline. Just as there have been Machine Learning breakthroughs in many other areas of applied science and business, it is also having a positive impact on quantitative investment.
Commentary on Machine Learning has recently hit new volumes, but Machine Learning is not a new subject. Indeed, it was in 1957 that Frank Rosenblatt invented the Perceptron – a machine that could learn to classify images – and today’s rapid developments in Machine Learning are built on three separate and long running revolutions:
- Computing power – this has broadly doubled every two years since the 1970s (Moore’s Law);
- Data generation, storage and retrieval – it’s estimated that 90% of the data in existence today were created in the last 2 years, whilst in 1981 a Gb of storage cost $300,000 today the price is below $0.101;
- Methodology – practical techniques from statistics, computer science, mathematics and engineering have matured and amalgamated into powerful new algorithms.
In recent months we have seen Google’s Machine Learning AlphaGo system beat South Korean Lee Sedol, one of the world’s most decorated Go players, by 4 games to 1. The game of Go is reported to have more possible board configurations than there are atoms in the universe. Given this, some commentators have asked whether the same computational fire-power2 can be recalibrated to tackle the “game” of investment. The reality is unlikely to be straightforward. Go has total observability and fixed clear rules, but with investment the “rules of the game” are more nebulous and are prone to change over time e.g. through regulatory, economic or demographic influence.
We think that an investment world of unbridled artificial intelligence with people rendered redundant is some way off. We do, however, think that machines will continue to enable investors to benefit from areas that the human brain struggles to reach. Homo sapiens individuals have strong pattern recognition ability over small homogeneous datasets but they struggle as the information set becomes larger and more varied. This is undoubtedly the case for financial information, which is not only burgeoning in size – AHL alone receives around 1.5bn data ticks every day – but is also very diverse, consisting of the obvious numbers and text, but also more unusual information sources. For example, in the case of energy and crop markets, important information may be found in meteorological diagrams and weather forecasts.
Machine Learning applied within quantitative finance offers a coherent, versatile and practical way of combining numerous and varied weak information sources into investment systems that have greater signalling power than any individual source. Such systems capture insights that both human intelligence and less sophisticated systematic models may miss. The data modelling challenges remain formidable, however, not least because financial datasets are vastly more noisy than those typical of the applications where Machine Learning has scored its greatest goals.
Image recognition using Deep Learning techniques, illustrating the potential of Machine Learning algorithms to recognise structure in complicated data sets. The algorithm was trained using an extremely large dataset of images. Left image: original picture, Centre: outline marked by human, Right: outline as determined by Machine Learning algorithm. A Machine Learning researcher in Man AHL worked on this project when previously employed as a Postdoctoral Research Fellow at the University of Oxford.
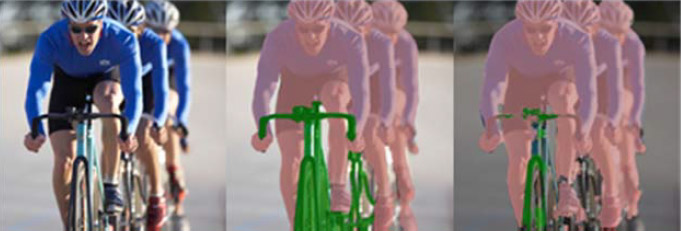
Source: Right-hand image produced using http://www.robots.ox.ac.uk/~szheng/crfasrnndemo
The Oxford-Man Institute (OMI) – with its focus on Machine Learning applied to quantitative investment – is at the forefront of academic Machine Learning research and is ideally placed, through its co-location with AHL’s research laboratory and the Department of Engineering Science’s Machine Learning Research Group, to collaborate with AHL’s research teams. The OMI also makes broad academic contributions across a range of disciplines, and solutions in one domain can resonate strongly in quantitative finance and investment. One such example is the Galaxy Zoo Supernovae project3 which presents online volunteers with astronomical images and asks them to classify what they see. Answers from the vast ensemble of volunteers are aggregated using Bayesian Machine Learning to determine which images contain supernovae. Collaboration between the OMI and AHL enabled the methodology for solving this astronomy problem to be applied to the task of extracting predictive signals from broker recommendations. Both applications involve classification decisions based on groups of potentially conflicting evidence where the abilities of the individual astronomers or analysts have to be learned and coherently combined. We expect to find similar examples where methodology is transferable across disciplines as our research efforts in Machine Learning increase and progress.
AHL has been researching Machine Learning techniques for around five years, and trading Machine Learning components within its multi-strategy client programmes since early 2014. The practical success we have obtained with these systems echoes the considerable experience of Professor Stephen Roberts (OMI Director, Royal Academy of Engineering / Man Group Research Chair in Machine Learning) and his 30-strong research group in other practical high-impact cases, e.g. in-flight monitoring of aerospace systems. The University’s Engineering Science Department – of which the OMI is formally part – has a broad portfolio of real world Machine Learning projects underway, including self-driving cars4 and computer vision5. In all these cases, there are clear implications for getting it wrong.
The Galaxy Zoo and image recognition examples are just two instances where AHL has been able to migrate applied Machine Learning research from academia and non-finance areas into its systematic investment research. The potential for Machine Learning to be used in AHL’s client programmes is vast, and we believe we are just starting-out on this journey. Through the partnership with Professor Roberts, his team at the OMI, and the wider expertise within the Department of Engineering, AHL is in a unique position to access cutting-edge research in the field, and intends to build-out its palette of machine learning across all of its investment vehicles.
Find out more
AHL Explains - Machine Learning
An Introduction to Statistical Learning, James, Witten, Hastie, Tibshirani, Springer (2013)
The Elements of Statistical Learning, Hastie, Tibshirani, Friedman, Springer (2009)
Deep Learning, Goodfellow, Bengio, Courville (2016)
1https://www.ibm.com/developerworks/community/files/form/anonymous/api/library/054c2ab9-ea33-4c70-b0c6-b5bb2482a098/document/7de665ff-2327-41a8-b7b0-5f0bba97356f/media/BIG%20DATA%20%2B%20MAINFRAME.pdf
http://notebooks.com/2011/03/09/hard-drive-prices-over-time-price-per-gb-from-1981-to-2010
2The deep learning approach used in AlphaGo contains a modern-day extension of artificial neural networks, which can in-turn be traced back to Rosenblatt’s seminal work
3See https://www.galaxyzoo.org/
4Robot Car: http://mrg.robots.ox.ac.uk/application/robotcar/
5Vision Group: http://www.robots.ox.ac.uk/~tvg/
You are now leaving Man Group’s website
You are leaving Man Group’s website and entering a third-party website that is not controlled, maintained, or monitored by Man Group. Man Group is not responsible for the content or availability of the third-party website. By leaving Man Group’s website, you will be subject to the third-party website’s terms, policies and/or notices, including those related to privacy and security, as applicable.