Introduction
The good old days are gone, and they’re never coming back.
Quite simply, the growth of quant investing means that portfolio management techniques which generated significant returns for discretionary managers a decade ago are now less effective. Strategies such as equity Value or Momentum have been systematised to the point where they can be sold as risk premia; discretionary managers now need to add alpha beyond factor returns to be successful. The propensity for information overload – via a maelstrom of bank research, research boutiques, social media, expert networks, data and news services – is ever growing. And the need for broad knowledge of the macro environment, as well as deep knowledge of specific securities has never been higher; as demonstrated by two very volatile years in which we have seen a pandemic-induced recession, supply chain havoc, inflation and a war in Europe.
The challenge has never been greater, which represents a significant opportunity for those managers with the skill to meet it head on.
So, the challenge has never been greater, which represents a significant opportunity for those managers with the skill to meet it head on. A deep knowledge of individual securities still provides ample scope for alpha generation. We believe that the growth of quantitative investing gives discretionary managers sources of new ideas and tools to enhance their investment process, allowing them to focus on the specialised knowledge that gives them their edge. From alpha research and alternative data to risk management and portfolio construction, incorporating quantitative techniques allows discretionary managers to thrive as never before.
The good old days may be gone. But the good new days have already arrived, bringing with them a new generation of alpha for discretionary managers.
Complementary Strengths
To successfully incorporate quantitative techniques into a discretionary portfolio, it is imperative to have a good understanding of the strengths of each school of asset management, and how they differ (Figure 1). As a rough rule of thumb, when compared with quantitative techniques, discretionary managers:
- Have a detailed knowledge of individual companies, carrying out research projects into a particular security;
- Have lower breadth, but a higher return, on individual bets;
- Are less reactive, but quicker to adapt, to changes in their investment environment.
In contrast, quantitative managers tend to:
- Conduct research into drivers of securities in general, rather than specific names;
- Trade thousands of names, and seek to aggregate incremental gains over a broad asset base to achieve returns;
- Follow an optimised approach to portfolio construction.
Figure 1. Discretionary and Quantitative Strengths and Weaknesses
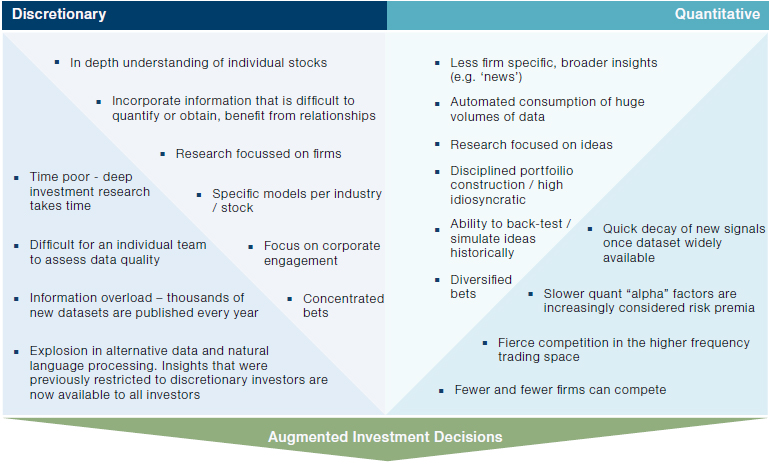
Source: Man GLG. For illustrative purpose only.
Over time, some of the unique advantages of both quant and discretionary managers have been eroded. On the discretionary side, areas of former strength such as the analysis of earnings calls transcripts have been automated by techniques such as natural language processing. From a quantitative perspective, as more and more quantitative techniques have entered the market, there has been evidence of alpha decay over time, especially in slower moving factor portfolios.
In our view, the nature of this erosion actually provides opportunity. The remaining areas of discretionary skill and quantitative alpha are essentially complementary: detailed knowledge of individual securities and an ability to predict future earnings revisions are not easily replicable from a quantitative perspective. Discretionary blind spots are very amenable to quantitative solutions which are concentrated in three areas: (1) collaborative alpha research and alternative data; (2) portfolio construction and active risk management; and (3) measuring and enhancing investment skill.
Collaborative Alpha Research and Alternative Data
Using quantitative techniques to analyse alternative data can help managers refine their conclusions, and improve their decision making.
High-quality fundamental research remains critical in discretionary investing and allows portfolio managers to develop a deeper understanding of a company’s prospect to gain an edge over their competitors. However, using quantitative techniques to analyse alternative data can help managers refine their conclusions, and improve their decision making. This is a collaborative process, which starts with an investment thesis generated by the portfolio manager. The quant team then assembles any relevant data, and backtests the idea against historical performance when feasible. The thesis can then be evaluated depending on the conclusiveness of the results, and possibly tweaked for further testing. Within the portfolio, ideas that are well supported by the research can be given a higher weighting. Furthermore, research can feed into the creation of automated screening tool to be used for future trades, which automatically identifies stocks with the relevant positive characteristics and reduces the legwork involved in portfolio construction.
Figure 2. Alpha Research – Collaboration Process
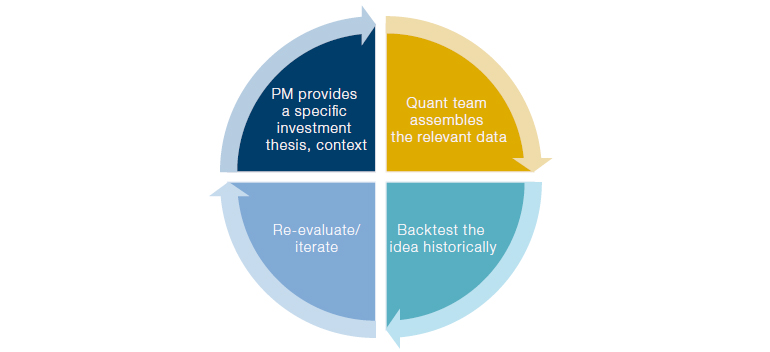
Source: Man GLG. For illustrative purposes only.
Indeed, the growth of alternative data has highlighted the need for a quant input into discretionary management. There is now vastly more data available than ever before, with the global production of data estimated to hit nearly 100 zettabytes in 2022 (Figure 3). For context, only two zettabytes were produced in 2010. Aggregating, sifting and analysing this data to produce genuine alpha is no mean feat – indeed simply sifting the wheat from the chaff is something of a herculean task.
Figure 3. Global Data Production
Problems loading this infographic? - Please click here
Source: IDC; Seagate; Statista estimates; as of May 2021. *Forecast for 2020 as of May 2021; forecast for 2021 to 2025 as of March 2021. Note: A zettabyte is equivalent to a trillion gigabytes.
But once the data is acquired, cleaned and analysed, how exactly does it enhance the investment process?
From a portfolio manager’s perspective, it can be used to support or defend an investment thesis. The classic example is credit card data, which can be used to observe sales for retail companies in real time. Analysis of such data can be a useful tool in helping analysts form a picture as to whether company guidance is likely to prove either too optimistic or pessimistic – and as a consequence, what the market reaction to earnings announcements may be. The spread between actual earnings and the prior analyst consensus is a significant driver of prices moves. Even major moves in earnings can do little to move security prices if they are anticipated by most investors. However, if alternative data is indicating that consensus is wrong, then portfolio managers are far better able to anticipate post-earnings price moves, which would otherwise catch them out. While alternative data on its own does not give a definitive answer, by using it in combination with traditional fundamental analysis, we have a much better chance of correctly predicting earnings – and therefore the stock price!
Aside from focusing on short-term prices movements, quantitative techniques can offer significant assistance in identifying long-term trends. By analysing alternative and traditional data in a quantitative manner, discretionary managers can spot emerging trends and identify those companies that are set to thrive over a 5-10 year window, anticipating significant future shifts in consumer behaviour. As an example, the pivot of multiple sports brands to become fashion brands in their own right was very clear to those analysing consumer spending data and gave a significant edge to those managers who understood the new earnings drivers of the relevant firms.
Alternative data reduces the number of blind spots surrounding single name stocks.
In addition, alternative data reduces the number of blind spots surrounding single name stocks. For instance, suppose a manager has a long position in a computer hardware seller. Its position in the industry ecosystem can tell us a great deal about its future performance. The earnings reports of its major suppliers can give us an indication about inventory purchasing, and how the target company projects its future sales. Likewise, the relationship of a firm to its competitors will be an indicator: if their earnings are highly correlated, we can get a sense of the firm’s future prospects from the general trend of industry revisions. By using alternative data to establish a precise view of the strength of different relationships within an industry in this way, portfolio managers can gain valuable insights, anticipating everything from future earnings to the erosion of a company’s competitive moat with much more accuracy.
The influence doesn’t all flow one way, however. The systematic signals produced by quant managers can also be significantly refined by the input of discretionary managers. While quant teams have access to broad data sets, honing in on specific key performance indicators which tend to influence discretionary investor decisions is something that is best done in tandem with discretionary managers. In this way, concentrated signals based on high accuracy modelling of KPIs can be built and traded, significantly enhancing the payoff for quant strategies. Likewise, signals can be reviewed by a discretionary specialist – be that sector, style, or region – as a sense check to ensure that the story of the data reflects an underlying economic reality rather than an anomaly.
Portfolio Construction & Active Risk Management
Quantitative techniques help focus the portfolio’s risk into areas in which managers have expertise.
The second area in which we feel that the addition of quant techniques can help with alpha generation for discretionary managers is in portfolio construction and risk management. Rather than originating investment theses, this focuses in extracting more alpha from existing ideas – i.e., plugging the bath rather than increasing the size of the taps, to use an analogy. Put simply, using these quantitative techniques helps focus the portfolio’s risk into areas in which managers have expertise – the companies themselves – and reduce unwanted risks that managers have no control over or has no view on, such as market risk and style risk. The net result is that the portfolio expresses the manager’s view in a much purer form.
Examples include the selection of the most appropriate risk model for the portfolio, establishing rules around the portfolio’s risk tolerance, or paying attention to hedging and trading strategies, managing factor and sector exposures to pre-set limits and limiting trades into already crowded names.
A good example of the latter scenario would be a short position in an ailing retailer, which currently trades as a Value stock. Assume the retailer is heavily shorted, and the portfolio is also short the Value factor on a net basis. If the stock’s volatility is increasing, a quantitative risk management process may advise the portfolio manager to cover the short. The chance of a short squeeze is relatively high, and the portfolio will still gain if Value does sell off. Even though the individual position still may be profitable, because of the potential short squeeze and the existing factor exposure the risk it represents to the downside means it is not worth keeping the position.
Application will vary according to the immediate needs of a portfolio. For instance, a portfolio manager might wish to tactically hedge the portfolio, cutting a specific factor exposure temporarily or to maintain a previous factor exposure after a block trade changes the tilt of the portfolio. Over a longer period, a systematic hedging strategy might aim to increase idiosyncratic risk within the portfolio or be used to run a long/short version of a long-only strategy. This latter goal would be achieved by identifying securities whose correlations to the long book allow them to act as a hedge. Quant analysis can also be used as sandbox to test new ideas. A good example would be factor hedging: by running new ideas alongside the existing strategy, portfolio managers can compare between the two, and ultimately see if the ‘real’ portfolio can be enhanced.
By using quant analysis to measure correlations more precisely, and build them into portfolio construction, discretionary managers can enhance performance.
It is worth stressing that none of this precision is possible without the use of quant techniques. Discretionary managers usually have a good idea of how their portfolio correlates to the economic cycle and various factors. Likewise, almost all portfolio managers have risk management teams, which often operate drawdown- and volatility-based rules around risk. However, as the old saying goes, if you can’t measure it, you can’t manage it. By using quant analysis to measure correlations more precisely, and build them into portfolio construction, discretionary managers can enhance performance.
However, it is still important to highlight that the application of quant techniques must take into account the constraints and preferences the discretionary portfolio manager is operating under. The end result should not be completely unrecognisable, but instead should bear an even closer resemblance to the intended risks the portfolio manager would like to be exposed to. Original security convictions and preferences around the degree of net exposure should be preserved: after all, the discretionary portfolio manager is there for a reason. Likewise, the portfolio should be comprehensible at a human scale – for instance, although a hedging basket can comprise up to hundreds of names, its overall features such as its aggregate beta or exposure to Momentum should be easily understood. With these caveats applied, we believe there is excellent scope to enhance the construction and risk management of discretionary portfolios with these techniques.
Measuring and Enhancing Investment Skill
Another way in which quantitative techniques can enhance discretionary investment is the insight it can give into what is actually driving portfolio returns. Not every money-making investment within a portfolio is equal. Some are the result of luck, others are driven by macro factors, random market moves and occasionally sheer exuberance. Others are the result of a consistent, repeatable process where an investment thesis is identified and then executed.
Once the manager is aware of their biases, steps can be taken to rectify them, which in turn can be used to create a high-performance culture.
Quantitative techniques can help differentiate one from the other by studying the underlying behaviours associated with a manager’s process through multiple lenses. These include a focus on the hit rate and average payoff of a portfolio manager’s ideas, measuring the counterfactual returns of the portfolio to test a manager’s skill at initiating and closing trades (i.e., checking what the return to a position would have been had the manager held onto it for longer, or closed it earlier). Not only can this be done on entry and exit, but counterfactuals can be used to test the performance of stop loss limits, the impact of increasing or reducing positions, and the effect of concentration risk and factor timing. Furthermore they can assist with checking the robustness of idea generation when outlier episodes of strong performance are artificially removed from the track record. By analysing these metrics, portfolio managers can gain a clearer understanding of how their decision making adds or detracts from returns. We have written about all this in more detail in Investing in Skill.
This process is also likely to highlight the human biases a portfolio manager is subject to. Once the manager is aware of their biases, steps can be taken to rectify them, which in turn can be used to create a high-performance culture where continuous improvement is the norm. If managers know how they are going wrong, they can take steps to improve their decision-making process, and hopefully make more correct decisions in the future. Likewise, would-be managers can be assessed in a scientific and data-driven fashion, which should ultimately improve the talent-spotting process.
Conclusion
We are entering the good new days for discretionary management, in which quantitative techniques are being used to enhance discretionary portfolios in a range of areas. From alpha generation, including the use of alternative data, to risk management, portfolio construction and performance analysis, the marriage of quant and discretionary management is underway – and reaping the rewards of both disciplines.
You are now leaving Man Group’s website
You are leaving Man Group’s website and entering a third-party website that is not controlled, maintained, or monitored by Man Group. Man Group is not responsible for the content or availability of the third-party website. By leaving Man Group’s website, you will be subject to the third-party website’s terms, policies and/or notices, including those related to privacy and security, as applicable.