We discuss the idea of adjacency, sports analytics, and how both sports and finance wrestle with the same issues when it comes to the interaction of human and machine.
We discuss the idea of adjacency, sports analytics, and how both sports and finance wrestle with the same issues when it comes to the interaction of human and machine.
December 2021
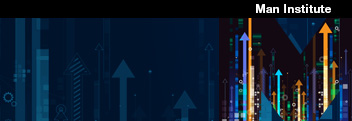
This article was originally published in Forbes on 18 November 2021.
Introduction
I’ve long been a fan of adjacency – the idea that by looking into cutting-edge thought in unfamiliar fields, we are able to drive breakthroughs closer to home. Man Group has a tie-up with Oxford University that seeks to take advantage of exactly that – while some researchers specifically look into how machine learning might be applied to the financial markets, the data scientists at the Oxford Man Institute are free to delve into everything from star mapping to measurement of tides. It’s amazing how many of the techniques they employ in their research are then adapted and applied to our own quantitative investment strategies. With cross-disciplinary research increasingly popular in academic fields and a broader recognition that blunt distinctions between STEM subjects and the humanities are reductive, it feels like adjacency theory is having a moment.
Every September, we host a client conference in Oxford and our line-up of speakers is guided by this idea of adjacency. Investors go to enough conferences where the subject matter stays firmly inside the rails of their working lives – we like the idea of sparking surprising connections by inviting speakers from other walks of life. This year, for instance, we had Peter Frankopan talking about ancient trade routes, Isla Finney speaking about chaos theory and the weather, Adam Kucharski showing how contagion theory links pandemics and gangland shootin gs, and Cade Massey recounting the past, present and future of quantitative approaches to athlete selection in sport.
Sports and Its Relevance to Finance
Each of these talks suggested parallels in finance, but there was a particular frisson during Massey’s presentation. Part of the excitement came from the fact that sports analytics is a wildly popular subject. I posted the graphic below on my LinkedIn a few weeks ago and watched as the views ticked up above 100,000. It illustrates the way that analytics has revolutionised the scoring patterns of basketball. Data showed that two-pointers attempted within the arc but outside the lane lines were the least efficient mode of scoring. If you were going to shoot from range, it was worth attempting a three-pointer or going for the greater certainty of a dunk or layup.
Figure 1. Top 200 Shot Locations in the NBA, 2001/02 Versus 2019/20
Source: Kirk Goldsberry. For illustrative purposes only.
Algorithms have dramatically changed the pattern of shot-taking in the NBA.
It wasn’t only the sports wonkery that got people’s pulses racing. It was the clear relevance of the subject to finance. This was a room in which many of the audience had deep knowledge of quantitative investing – the use of algorithms to guide investment decisions. As Massey walked through the evolution of quantitative approaches to making decisions about athlete selection – the data revolution outlined in Michael Lewis’s book Moneyball (later made into a film with Brad Pitt) – you could see eyes lighting up in recognition. The financial markets, you see, went through a very similar evolution when the possibilities of algorithmic investment became apparent in the early years of the century.
What’s more, it feels as if both sports and finance spend their time wrestling with many of the same issues when it comes to the interaction of human and machine. How do you ensure that models are delivering the right outputs? How do you know if a model’s underperformance is the sign of a terminal flaw, a glitch, or just part of the cycle? Which areas are humans still able to do better than computers? How do you ensure that you’re inputting the correct data to your models? Massey, a Professor at Wharton as well as the co-creator of the Massey-Peabody NFL Power Rankings for the Wall Street Journal, spoke about how each of these issues was addressed by major baseball and (American) football franchises, and how quantitative strategies are gradually spreading to other sports, from soccer to cricket.
Culture Versus Analytic Tractability
I kept in touch with Massey after the event and thought it would be interesting to speak to him, in particular teasing out the elements that seemed to have the greatest relevance for those of us in finance. I began by asking him why some sports had been swifter to adopt quant strategies than others – basketball and baseball were way ahead of soccer, for instance. Massey spoke about two key factors – analytical tractability (how easy the data are to separate and manipulate) and culture. “Individual performance in baseball is almost perfectly independent,” he said, “unlike any other major sport. This greatly simplifies all analysis. And soccer lags because of tractability.” Not only is performance highly interdependent in soccer, but there are 22 players on the pitch at one time (versus 10 in basketball or 12 in hockey). And play is continuous (unlike American football) so naturally occurring data, ready-made for analysis, are rare.
The other main factor is culture. “Analytics spread in basketball far faster than in any other sport because the culture of the sport, and of many individual franchises, is less traditional than in other sports,” Massey told me. “One of the main reasons for this is the influx of new owners, many of whom came from finance and tech backgrounds, two industries heavily invested in analytics. And ownership is the key determinant of how a sports franchise operates.”
Figure 2 outlines these two central concepts that define the impact of analytics on sport.
Figure 2. Culture Versus Analytic Tractability
Source: Cade Massey. For illustrative purposes only.
For sports to embrace quant strategies, they need an open culture and easy-to-manipulate data
The applicability of this analysis to the investment industry ought to be clear. You need to encourage a culture within a firm that is open to the benefits of machine learning and algorithmic approaches to investment. This often means a process of education and demystification, an explanation that computers augment, rather than replace, our human decision-making processes.
As far as analytical tractability goes, this is really a question of the frequency, quality and availability of data. The reason that quant credit, for instance, has lagged behind quant equity (although it is fast catching up) is simply that there are fewer visible trading data and a murkier history of data to analyse. Quant strategies can be applied to all asset classes but there needs to be sufficient high-quality data to use as the foundation for models.
Massey also spoke about the interaction of human and machine. After the Moneyball upheaval driven by Billy Beane at the Oakland Athletics in baseball and Daryl Morey at the Houston Rockets in basketball, others felt that they had to race into data-driven athlete selection. Every revolution overshoots its initial goals, and some teams almost entirely discarded the human element from the process of athlete selection, a situation which led to some bad decisions being made. There is now a recognition, Massey told me, that you need to think about a continuum that runs between “augmented expert” and “supervised algorithm”, with neither extreme – unsupervised algorithm or machinefree expertise – being advisable.
This is also about culture, Massey said. “The key factor in getting the blend right is having members of the group more committed to improving decision-making than to being right. A group that shares a common identity instead of membership in factions. A culture that says this process is so hard that no one individual or faction could possibly have a monopoly on the correct perspective.” There are certain areas where machines will perform more efficiently, others where humans will do better. Figure 3 shows the history of the interaction between experts and algorithms, and the ideal mix, which allocates strategic decisions to experts, mechanical decisions to algorithms, and has a broad swathe of activities where there is collaboration between human and machine. Still, according to Massey, the leading clubs are allocating too many processes to computers without human intervention.
Figure 3. History of Interaction Between Experts and Algorithms, and the Ideal Mix
Source: Cade Massey. For illustrative purposes only.
The best teams allocate responsibilities between algorithms and humans, with most spheres seeing some element of collaboration between the two.
In finance, we know what happens when algorithms are allowed to operate independently of expert supervision – the Flash Crash of 2010 is just the most highprofile example of numerous times when the machines got ahead of the people operating them. Massey recognises the parallel.
We need to think of our interaction with machine learning as an ongoing process, he says. “There does seem to be an evolutionary direction, from enhanced expert to supervised algorithm. It’s useful to think of it as we do skill acquisition, in general. When we first learn a new skill, we have to do everything consciously. Over time, we gain facility with the fundamental behaviours and begin doing them automatically, without much if any conscious thought. This is great because then we can turn our attention to higher-level matters. A good model, in an organisation with a healthy attitude toward models, should evolve over time and that evolution should subsume expert input. The expert input improves the model to the point that, eventually, that particular input can be automated. Expert attention can then be redirected to more value-added tasks.”
A useful way of thinking about the process of integrating algorithms and data analytics into your world, whether you run a hedge fund or a professional sports team.
You are now exiting our website
Please be aware that you are now exiting the Man Institute | Man Group website. Links to our social media pages are provided only as a reference and courtesy to our users. Man Institute | Man Group has no control over such pages, does not recommend or endorse any opinions or non-Man Institute | Man Group related information or content of such sites and makes no warranties as to their content. Man Institute | Man Group assumes no liability for non Man Institute | Man Group related information contained in social media pages. Please note that the social media sites may have different terms of use, privacy and/or security policy from Man Institute | Man Group.